Important Announcement
Please join the DSML Slack workspace to facilitate offline discussion.
Workshop Program - Monday, 29 June 2020
15:00 CET |
Welcome to DSN-DSML 2020
Homa Alemzadeh, University of Virginia
Video
|
|
Session 1: Keynote Talk
Session Chair: Karthik Pattabiraman, University of British Columbia
|
15:05 CET
15:35 CET
|
Interpretability-Driven Dependable and Secure Machine Learning
Michael Lyu, Chinese University of Hong Kong
Video
Q&A
|
15:45 CET |
Break |
|
Session 2: Attacks
Session Chair: Varun Chandrasekaran, University of Wisconsin-Madison
|
15:55 CET
16:10 CET
16:25 CET
|
TAaMR: Targeted Adversarial Attack against Multimedia Recommender Systems
Tommaso Di Noia, Daniele Malitesta, Felice Antonio Merra
Video
On The Generation Of Unrestricted Adversarial Examples
Mehrgan Khoshpasand, Ali Ghorbani
Video
Blackbox Attacks on Reinforcement Learning Agents Using Approximated Temporal Information
Yiren Zhao, Ilia Shumailov, Han Cui, Xitong Gao, Robert Mullins, Ross Anderson
Video
|
16:40 CET |
Break |
|
Session 3: Validation, Verification, and Defense
Session Chair: Florian Tramèr, Stanford University
|
16:50 CET
17:05 CET
17:20 CET
|
PyTorchFI: A Runtime Perturbation Tool for DNNs
Abdulrahman Mahmoud, Neeraj Aggarwal, Alex Nobbe, Jose Rodrigo Sanchez Vicarte, Sarita Adve, Christopher W. Fletcher, Iuri Frosio, Siva Kumar Sastry Hari
Video
Online Verification through Model Checking of Medical Critical Intelligent Systems
João Martins, Raul Barbosa, Nuno Lourenço, Jacques Robin, Henrique Madeira
Video
BlurNet: Defense by Filtering the Feature Maps
Ravi Raju, Mikko Lipasti
Video
|
17:35 CET |
Break |
|
Session 4: Keynote Talk
Session Chair: Nicolas Papernot, University of Toronto & Vector Institute
|
17:45 CET
18:15 CET
|
Using Secure AI to Secure Real Users (435M of them)
Rajarshi Gupta, Avast Security
Video
Q&A
|
18:25 CET |
Discussion and Closing |
|
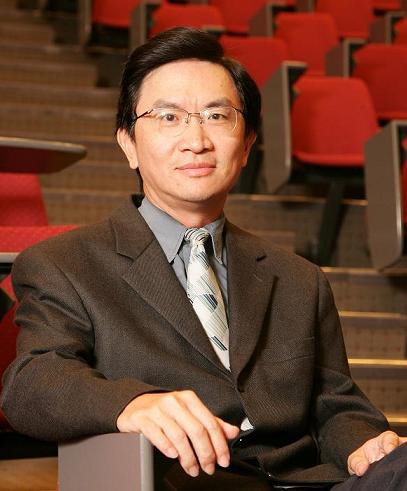
Interpretability-Driven Dependable and Secure Machine Learning
Michael Lyu, Chinese University Of Hong Kong
Abstract:
Although artificial intelligence has advanced the state-of-the-art in many domains, its interpretability,
dependability, and security remain unsatisfactory, hindering the rapid deployment in many safety-critical scenarios.
Among these characteristics, interpretability is at the core since the human trust builds upon the interpretability
of model predictions and understanding of unexpected behaviors (e.g., error predictions, adversarial attacks).
In this talk, I will introduce some of our recent investigations on model interpretability in both natural
language processing and computer vision domains. Besides, I will illustrate our recent attempts on
dependable and secure machine learning from the interpretability perspective.
Finally, I will share some thoughts on the related research directions.
Speaker Bio: Michael R. Lyu is a Professor and the Chairman in the Computer Science & Engineering Department at the
Chinese University of Hong Kong. He received a B.S. in Electrical Engineering from the National Taiwan University,
an M.S. in Electrical and Computer Engineering from University of California, Santa Barbara, and a Ph.D.
in Computer Science from University of California, Los Angeles. His research interests include software
reliability engineering, dependable computing, machine learning, artificial intelligence, and distributed systems.
He published a widely cited McGraw-Hill Handbook of Software Reliability Engineering, and a Wiley book on
Software Fault Tolerance. He is a Fellow of the IEEE, a Fellow of ACM, a Fellow of AAAS, and an
IEEE Reliability Society Engineer of the Year. He also appears in The AI
2000 Most Influential Scholars Annual List in 2020.
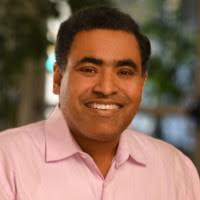
Using Secure AI to Secure Real Users (435M of them)
Rajarshi Gupta, Avast
Abstract: Recent years have seen heavy utilization of AI in security,
but the complexities of a massively scalable production-quality security pipeline is often hard to grasp.
In this seminar, we will discuss state-of-the-art AI techniques used to deter daily attacks,
by drawing from experience of protecting 435M users (across PCs, mobiles, IoTs) at Avast.
We will also identify gaps that exist between academic research in AI-Security,
and the daily challenges of real-world attacker-defender contests.
Finally, we suggest ways to bridge those gaps,
to make the academic research more viable and valuable in real deployments.
Speaker Bio: Rajarshi Gupta is the Head of AI at Avast Software, the largest consumer security companies in the world.
He has a PhD in EECS from UC Berkeley and has built a unique expertise at the intersection of Artificial Intelligence,
Cybersecurity and Networking.
Prior to joining Avast, Rajarshi worked for many years at Qualcomm Research, where he created ‘Snapdragon Smart Protect’,
the first ever product to achieve On-Device Machine Learning for Security.
Rajarshi has authored over 200 issued U.S. Patents, and is featured on the wikipedia page for most prolific inventors in history.
|
|